Edward Austin is Senior research associate at the Department of Mathematics and Statistics at Lancaster University, UK.
Edward Austin: Detecting Emergent Anomalies in Functional Data
This talk will focus on recent work about the sequential detection of anomalies within partially observed functional data, motivated by a problem encountered by an industrial collaborator. Classical sequential changepoint detection approaches look for changes in the parameters, or structure, of a data sequence and are not equipped to handle the complex non-stationarity and dependency structure of functional data. Conversely, existing functional data approaches require the full observation of the curve before anomaly detection can take place. We propose a new method, FAST, that performs sequential detection of anomalies in partially observed functional data. This talk will introduce the approach, and some associated theoretical results, and highlight its application on telecommunications data.
This is joint work with Idris Eckley and Lawrence Bardwell.
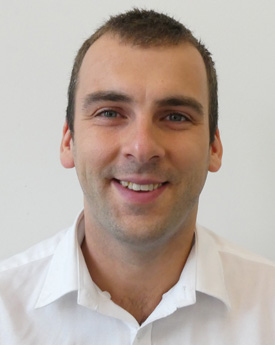